Live Session
Session 16: Large Language Models 2
Reproducibility
A Comparative Analysis of Text-Based Explainable Recommender Systems
Alejandro Ariza-Casabona (University of Barcelona), Ludovico Boratto (University of Cagliari) and Maria Salamo (Departament of Mathematics and Computer Science, Universitat de Barcelona)
Abstract
One way to increase trust among users towards recommender systems is to provide the recommendation along with a textual explanation. In the literature, extraction-based, generation-based, and, more recently, hybrid solutions based on retrieval-augmented generation have been proposed to tackle the problem of text-based explainable recommendation. However, the use of different datasets, preprocessing steps, target explanations, baselines, and evaluation metrics complicates the reproducibility and state-of-the-art assessment of previous work among different model categories for successful advancements in the field. Our aim is to provide a comprehensive analysis of text-based explainable recommender systems by setting up a well-defined benchmark that accommodates generation-based, extraction-based, and hybrid approaches. Also, we enrich the existing evaluation of explainability and text quality of the explanations with a novel definition of feature hallucination. Our experiments on three real-world datasets unveil hidden behaviors and confirm several claims about model patterns. Our source code and preprocessed datasets are available at https://anonymous.4open.science/r/text-exp-recsys24/.
Join the Conversation
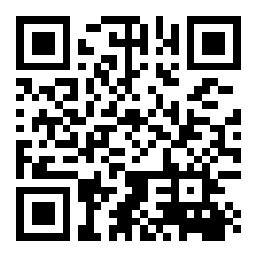