Live Session
Session 14: Robust RecSys 2
Main Track
Accelerating the Surrogate Retraining for Poisoning Attacks against Recommender Systems
Yunfan Wu (Institute of Computing Technology, Chinese Academy of Sciences), Qi Cao (Institute of Computing Technology, Chinese Academy of Sciences), Shuchang Tao (Institute of Computing Technology, Chinese Academy of Sciences), Kaike Zhang (Institute of Computing Technology, Chinese Academy of Sciences), Fei Sun (Institute of Computing Technology, Chinese Academy of Sciences) and Huawei Shen (Institute of Computing Technology, Chinese Academy of Sciences)
Abstract
Recent studies have demonstrated the vulnerability of recommender systems to data poisoning attacks, where adversaries inject carefully crafted fake user interactions into the training data to manipulate recommendations for target items.Current attack methods involve iterative retraining a surrogate recommender on the poisoned data with latest fake users to optimize the attack. However, this repetitive retraining is highly time-consuming, hindering the efficient assessment and optimization of fake users. To mitigate this computational bottleneck and develop a more effective attack in an affordable time, we analyze the retraining process and find that a change in the representation of one user/item will cause a cascading effect through the user-item interaction graph. Under theoretical guidance, we introduce Gradient Passing (GP), a novel technique that explicitly passes gradients between interacted user-item pairs during backpropagation, thereby approximate the cascading effect and accelerating retraining. With just a single training step, GP can achieve effects comparable to multiple original steps. Under the same number of retraining epochs, GP enables a closer approximation of the surrogate recommender to the victim. This improved approximation provides better guidance for optimizing fake users, ultimately leading to enhanced data poisoning attacks. Extensive experiments on real-world datasets demonstrate the efficiency and effectiveness of our proposed GP.
Join the Conversation
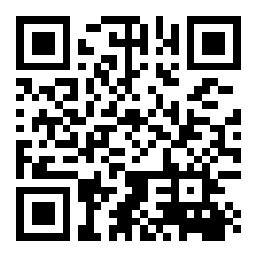