Live Session
Session 2: Bias and Fairness 1
Reproducibility
AMBAR: A dataset for Assessing Multiple Beyond-Accuracy Recommenders
Elizabeth Gómez (Universitat de Barcelona), David Contreras (Universidad Arturo Prat), Ludovico Boratto (University of Cagliari) and Maria Salamo (Departament of Mathematics and Computer Science, Universitat de Barcelona)
Abstract
Nowadays a recommendation model should exploit additional information from both the user and item perspectives, in addition to utilizing user-item interaction data. Datasets are central in offering the required information for evaluating new models or algorithms. Although there are many datasets in the literature with user and item properties, there are several issues not covered yet: (i) it is difficult to perform cross-analysis of properties at user and item level as they are not related in most cases; and (ii) on top of that, in many occasions datasets do not allow analysis at different granularity levels. In this paper, we propose a new dataset in the music domain, named AMBAR, that includes the above-mentioned issues. Besides detailing in depth the structure of the new dataset, we also show its application in contexts (i.e., multi-objective, fair, and calibrated recommendations) where both the effectiveness and the beyond-accuracy perspectives of recommendation are assessed.
Join the Conversation
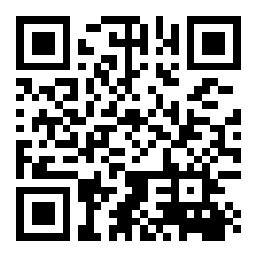