Live Session
Wednesday Posters
Research
CAPRI-FAIR: Integration of Multi-sided Fairness in Contextual POI Recommendation Framework
Francis Dela Cruz (University of New South Wales), Flora D. Salim (University of New South Wales), Yonchanok Khaokaew (University of New South Wales) and Jeffrey Chan (RMIT University)
Abstract
Point-of-interest (POI) recommendation, a form of context-aware recommendation, takes into account spatio-temporal constraints and contexts like distance, peak business hours, and previous user check-ins. Given the ability of these kinds of systems to influence not just the consumer's travel experience, but also the POI's business, it is important to consider fairness from multiple perspectives. Unfortunately, these systems tend to provide less accurate recommendations to inactive users, and less exposure to unpopular POIs. The goal of this paper is to develop a post-filter methodology that incorporates provider and consumer fairness factors into pre-existing recommendation models, to satisfy fairness metrics like item exposure, and performance metrics like precision and distance, making the system more sustainable to both consumers and providers. Experiments have shown that using a linear scoring model for provider fairness in re-scoring recommended items yields the best tradeoff between performance and long-tail exposure, in some cases without a significant decrease in precision. When attempting to address consumer fairness by recommending more popular POIs to inactive users, the result was an increase in precision for only some recommendation models and datasets. Finally, when considering the tradeoff between both parameters, the combinations that reached the Pareto front of consumer and provider fairness, unfortunately, achieved the lowest precision values. We find that the nature of this tradeoff depends heavily on the model and the dataset.
Join the Conversation
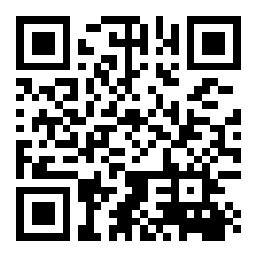