Live Session
Wednesday Posters
Research
Comparative Analysis of Pretrained Audio Representations in Music Recommender Systems
Yan-Martin Tamm (University of Tartu) and Anna Aljanaki (University of Tartu)
Abstract
Over the years, Music Information Retrieval (MIR) has proposed various foundation models pretrained on large amounts of music data. Transfer learning showcases proven effectiveness of foundation models with a broad spectrum of downstream tasks, including auto-tagging and genre classification. However, MIR papers generally do not explore the efficiency of foundation models for Music Recommender Systems (MRS). In addition, the Recommender Systems (RS) community tends to favour traditional end-to-end neural network learning over these models. Our research addresses this gap and evaluates the applicability of six pretrained foundation models (MusicFM, Music2Vec, MERT, EncodecMAE, Jukebox, and MusiCNN) in the context of MRS. We assess their performance using three recommendation models: K-nearest neighbours (KNN), shallow neural network, and BERT4Rec. Our findings suggest that these models exhibit significant performance variability between traditional MIR tasks and MRS, indicating that valuable aspects of musical information captured by foundation models may differ depending on the task. This study establishes a foundation for further exploration of pretrained foundation models to enhance music recommendation systems.
Join the Conversation
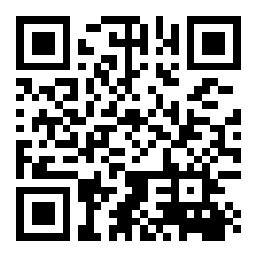