Live Session
Tuesday Posters
Research
Δ-OPE: Off-Policy Estimation with Pairs of Policies
Olivier Jeunen (ShareChat) and Aleksei Ustimenko (ShareChat)
Abstract
The off-policy paradigm casts recommendation as a counterfactual decision-making task, allowing practitioners to unbiasedly estimate online metrics using offline data. This leads to effective evaluation metrics, as well as learning procedures that directly optimise online success. Nevertheless, the high variance that comes with unbiased- ness is typically the crux that complicates practical applications. An important insight is that the difference between policy values can often be estimated with significantly reduced variance, if said policies have positive covariance. This allows us to formulate a pairwise off-policy estimation task: Δ-OPE. Δ-OPE subsumes the common use-case of estimating improve- ments of a learnt policy over a production policy, using data col- lected by a stochastic logging policy. We introduce Δ-OPE methods based on the widely used Inverse Propensity Scoring estimator and its extensions. Moreover, we characterise a variance-optimal additive control variate that further enhances efficiency. Simulated, offline, and online experiments show that our methods significantly improve performance for both evaluation and learning tasks.
Join the Conversation
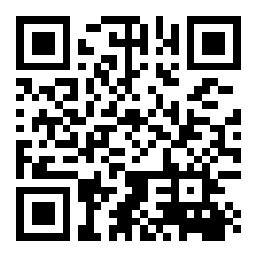