Live Session
Thursday Posters
Research
Does It Look Sequential? An Analysis of Datasets for Evaluation of Sequential Recommendations
Anton Klenitskiy (Sber AI Lab), Anna Volodkevich (Sber AI Lab), Anton Pembek (Sber AI Lab, Lomonosov Moscow State University (MSU)) and Alexey Vasilev (Sber AI Lab)
Abstract
Sequential recommender systems are an important and demanded area of research. Such systems aim to use the order of interactions in a user’s history to predict future interactions. The premise is that the order of interactions and sequential patterns play an important role. Therefore, it is crucial to use datasets that exhibit a sequential structure for a proper evaluation of sequential recommenders.We apply several methods based on the random shuffling of the user's sequence of interactions to assess the strength of sequential structure across 15 datasets, frequently used for sequential recommender systems evaluation in recent research papers presented at top-tier conferences. As shuffling explicitly breaks sequential dependencies inherent in datasets, we estimate the strength of sequential patterns by comparing metrics for shuffled and original versions of the dataset. Our findings show that several popular datasets have a rather weak sequential structure.
Join the Conversation
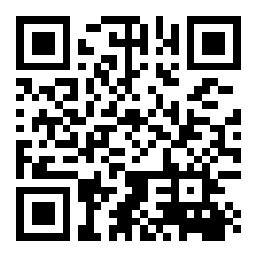