Live Session
Thursday Posters
Industry Poster
Enhancing Performance and Scalability of Large-Scale Recommendation Systems with Jagged Flash Attention
Rengan Xu (Meta), Junjie Yang (Meta), Yifan Xu (Meta), Hong Li (Meta), Xing Liu (Meta), Devashish Shankar (Meta), Haoci Zhang (Meta), Meng Liu (Meta), Boyang Li (Meta), Yuxi Hu (Meta), Mingwei Tang (Meta), Zehua Zhang (Meta), Tunhou Zhang (Meta), Dai Li (Meta), Sijia Chen (Meta), Jiaqi Zhai (Meta), Bill Zhu (Meta), Arnold Overwijk (Meta) and Sri Reddy (Meta)
Abstract
The integration of hardware accelerators has significantly advanced the capabilities of modern recommendation systems, enabling the exploration of complex ranking paradigms previously deemed impractical. However, the GPU-based computational costs present substantial challenges. In this paper, we demonstrate our development of an efficiency-driven approach to explore these paradigms, moving beyond traditional reliance on native PyTorch modules. We address the specific challenges posed by ranking models’ dependence on categorical features, which vary in length and complicate GPU utilization. We introduce Jagged Feature Interaction Kernels, a novel method designed to extract fine-grained insights from long categorical features through efficient handling of dynamically sized tensors. We further enhance the performance of attention mechanisms by integrating Jagged tensors with Flash Attention. As the feature length grows, the Jagged Flash Attention is able to scale memory linearly rather than quadratically. Our experimental results demonstrate that Jagged Flash Attention achieves speedups of 2.4× to 5.6× over dense attention and reduces memory usage by up to 21.8×. This allows to scale the recommendation systems with longer features and more complex model architecture.
Join the Conversation
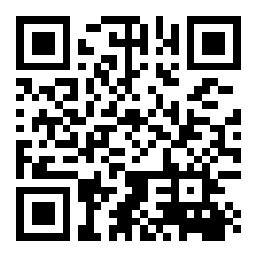