Live Session
Session 3: Bias and Fairness 2
Main Track
Fair Reciprocal Recommendation in Matching Markets
Yoji Tomita (CyberAgent, Inc.) and Tomohiko Yokoyama (The University of Tokyo)
Abstract
Recommender systems play an increasingly crucial role in shaping people’s opportunities, particularly in online dating platforms. It is essential from the user’s perspective to increase the probability of matching with a suitable partner while ensuring an appropriate level of fairness in the matching opportunities. We investigate reciprocal recommendation in two-sided matching markets between agents divided into two sides (e.g., men and women). We assume that a successful match requires mutual interest from both men and women involved, and agents would desire to be positioned favorably in the others’ the recommendation lists. We define each agent’s opportunity to be recommended and introduce its fairness criterion, envy-freeness, from the perspective of the fair division theory. The recommendations that approximately maximize the expected number of matches between men and women, empirically obtained by heuristic algorithms, are likely to result in significant unfairness of opportunity. Therefore, there can be a trade-off between maximizing the expected matches and ensuring fairness of opportunity. We propose a method to find a policy that is close to being envy-free by leveraging the Nash social welfare function. Experiments on synthetic and a real-world dataset demonstrate the effectiveness of our approach in achieving both relatively high expected matches and fairness for opportunities of both men and women in reciprocal recommender systems.
Join the Conversation
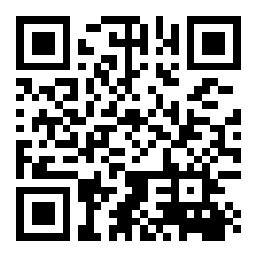