Live Session
Doctoral Symposium
Doctoral Symposium
Fairness explanation in recommender systems
Luan Souza (University of São Paulo)
Abstract
Fairness in recommendations is an emerging area in recommender systems, aiming to mitigate discriminations against individuals or/and groups of individuals in recommendations. These mitigation strategies rely on statistical bias detection, which is a non-trivial task that requires complex analysis and interventions to ensure fairness in these engines. Furthermore, fairness interventions in recommender systems involve a tradeoff between fairness and performance of the recommendation lists, impacting the user experience with less accurate lists. In this context, fairness interventions with explanations have been proposed recently, mitigating discrimination in recommendation lists and providing explainability about the recommendation process and the impact of the fairness interventions. However, in spite of the different approaches it is still not clear how these proposals compare with each other, even those that propose to mitigate the same kind of bias. In addition, the contribution of these different explainable algorithmic fairness approaches to users’ fairness perceptions was not explored until the moment. Looking at these gaps, our doctorate project aims to investigate how these explainable fairness proposals compare to each other and how they are perceived by the users, in order to identify which fairness interventions and explanation strategies are most promising to increase transparency and fairness perceptions of recommendation lists.
Join the Conversation
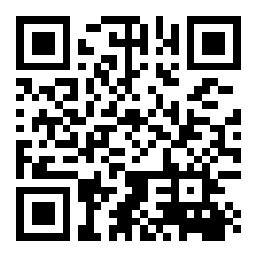