Live Session
Wednesday Posters
Research
Fairness Matters: A look at LLM-generated group recommendations
Antonela Tommasel (ISISTAN Research Institute, CONICET-UNCPBA)
Abstract
Recommender systems play a crucial role in how users consume information, with group recommendation receiving considerable attention. Ensuring fairness in group recommender systems entails providing recommendations that are useful and relevant to all group members rather than solely reflecting the majority’s preferences, while also addressing fairness concerns related to sensitive attributes (e.g., gender). Recently, the advancements on Large Language Models (LLMs) have enabled the development of new kinds of recommender systems. However, LLMs can perpetuate social biases present in training data, posing risks of unfair outcomes and harmful impacts. We investigated LLMs impact on group recommendation fairness, establishing and instantiating a framework that encompasses group definition, sensitive attribute combinations, and evaluation methodology. Our findings revealed the interactions patterns between sensitive attributes and LLMs and how they affected recommendation. This study advances the understanding of fairness considerations in group recommendation systems, laying the groundwork for future research.
Join the Conversation
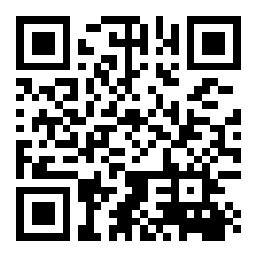