Live Session
Session 14: Robust RecSys 2
Main Track
FedLoCA: Low-Rank Coordinated Adaptation with Knowledge Decoupling for Federated Recommendations
Yuchen Ding (University of Science and Technology of China), Siqing Zhang (University of Science and Technology of China), Boyu Fan (University of Helsinki), Wei Sun (University of Science and Technology of China), Yong Liao (University of Science and Technology of China) and Pengyuan Zhou (Aarhus University)
Abstract
Privacy protection in recommendation systems is gaining increasing attention, for which federated learning has emerged as a promising solution. Current federated recommendation systems grapple with high communication overhead due to sharing dense global embeddings, and also poorly reflect user preferences due to data heterogeneity. To overcome these challenges, we propose a two-stage Federated Low-rank Coordinated Adaptation (FedLoCA) framework to decouple global and client-specific knowledge into low-rank embeddings, which significantly reduces communication overhead while enhancing the system’s ability to capture individual user preferences amidst data heterogeneity. Further, to tackle gradient estimation inaccuracies stemming from data sparsity in federated recommendation systems, we introduce an adversarial gradient projected descent approach in low-rank spaces, which significantly boosts model performance while maintaining robustness. Remarkably, FedLoCA also alleviates performance loss even under the stringent constraints of differential privacy. Extensive experiments on various real-world datasets demonstrate that FedLoCA significantly outperforms existing methods in both recommendation accuracy and communication efficiency.
Join the Conversation
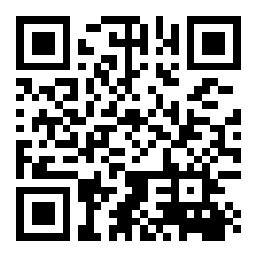