Live Session
Session 16: Large Language Models 2
Main Track
FLIP: Fine-grained Alignment between ID-based Models and Pretrained Language Models for CTR Prediction
Hangyu Wang (Shanghai Jiao Tong University), Jianghao Lin (Shanghai Jiao Tong University), Xiangyang Li (Huawei Noah’s Ark Lab), Bo Chen (Huawei Noah’s Ark Lab), Chenxu Zhu (Huawei Noah’s Ark Lab), Ruiming Tang (Huawei Noah’s Ark Lab), Weinan Zhang (Shanghai Jiao Tong University) and Yong Yu (Shanghai Jiao Tong University)
Abstract
Click-through rate (CTR) prediction plays as a core function module in various personalized online services. The traditional ID-based models for CTR prediction take as inputs the one-hot encoded ID features of tabular modality, which capture the collaborative signals via feature interaction modeling. But the one-hot encoding discards the semantic information included in the textual features. Recently, the emergence of Pretrained Language Models (PLMs) has given rise to another paradigm, which takes as inputs the sentences of textual modality obtained by hard prompt templates and adopts PLMs to extract the semantic knowledge. However, PLMs often face challenges in capturing field-wise collaborative signals and distinguishing features with subtle textual differences. In this paper, to leverage the benefits of both paradigms and meanwhile overcome their limitations, we propose to conduct Fine-grained feature-level ALignment between ID-based Models and Pretrained Language Models (FLIP) for CTR prediction. Unlike most methods that solely rely on global views through instance-level contrastive learning, we design a novel jointly masked tabular/language modeling task to learn fine-grained alignment between tabular IDs and word tokens. Specifically, the masked data of one modality (\ie, IDs and tokens) has to be recovered with the help of the other modality, which establishes the feature-level interaction and alignment via sufficient mutual information extraction between dual modalities. Moreover, we propose to jointly finetune the ID-based model and PLM by adaptively combining the output of both models, thus achieving superior performance in downstream CTR prediction tasks. Extensive experiments on three real-world datasets demonstrate that FLIP outperforms SOTA baselines, and is highly compatible with various ID-based models and PLMs.The code is available for reviewers\footnote{\url{https://anonymous.4open.science/r/FLIP-2534}}.
Join the Conversation
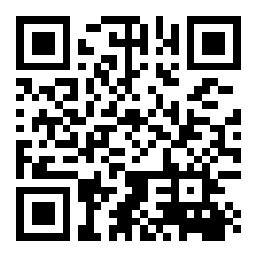