Live Session
Session 10: Graph Learning
Main Track
Information-Controllable Graph Contrastive Learning for Recommendation
Zirui Guo (Beijing University of Posts and Telecommunications), Yanhua Yu (Beijing University of Posts and Telecommunications), Yuling Wang (Beijing University of Posts and Telecommunications), Kangkang Lu (Beijing University Of Posts and Telecommunications), Zixuan Yang (Beijing University Of Posts and Telecommunications), Liang Pang (Institute of Computing Technology, Chinese Academy of Sciences) and Tat-Seng Chua (National University of Singapore)
Abstract
In the evolving landscape of recommender systems, Graph Contrastive Learning (GCL) has become a prominent method for enhancing recommendation performance by alleviating the issue of data sparsity. However, existing GCL-based recommendations often overlook the control of shared information between the contrastive views. In this paper, we initially analyze and experimentally demonstrate these methods often lead to the issue of augmented representation collapse, where the representations between views become excessively similar, diminishing their distinctiveness. To address this issue, we propose the Information-Controllable Graph Contrastive Learning (IGCL) framework, a novel approach that focuses on optimizing the shared information between views to include as much relevant information for the recommendation task as possible while maintaining an appropriate level. In particular, we design the Collaborative Signals Enhanced Augmentation module to infuse the augmented representation with rich, task-relevant collaborative signals. Furthermore, the Information-Controllable Contrastive Learning module is designed to direct control over the magnitude of shared information between the contrastive views to avoid over-similarity. Extensive experiments on three public datasets demonstrate the effectiveness of IGCL, showcasing significant improvements in performance and the capability to alleviate augmented representation collapse.
Join the Conversation
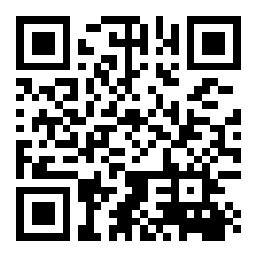