Live Session
Session 5: Cross-domain and Cross-modal Learning
Main Track
Instructing and Prompting Large Language Models for Explainable Cross-domain Recommendations
Alessandro Petruzzelli (University of Bari Aldo Moro), Cataldo Musto (University of Bari Aldo Moro), Lucrezia Laraspata (University of Bari Aldo Moro), Ivan Rinaldi (University of Bari Aldo Moro), Marco de Gemmis (University of Bari Aldo Moro), Pasquale Lops (University of Bari Aldo Moro) and Giovanni Semeraro (University of Bari Aldo Moro)
Abstract
In this paper, we present a strategy to provide users with explainable cross-domain recommendations (CDR) that exploits large language models (LLMs). Generally speaking, cross-domain recommender systems typically suffer of data sparsity issues, since they require a large amount of data labeled in both base and target domains, which are not easy to collect. Accordingly, our approach relies on the intuition that the knowledge that is already encoded in LLMs can be used to bridge the domains and seamlessly provide users with personalized cross-domain suggestions. To this end, we designed a pipeline to: (a) instruct a LLM to handle a CDR task; (b) design a personalized prompt, based on the preferences of the user in a base domain, in both zero-shot and one-shot settings; (c) feed the LLM with the prompt, and process the answer in order to extract the recommendations in a target domain, together with a natural language explanation supporting the suggestion. As shown in the experimental evaluation, our approach beats several established state-of-the-art baselines for CDR in most of the experimental settings, thus showing the effectiveness of LLMs also in this novel and scarcely investigated scenario.
Join the Conversation
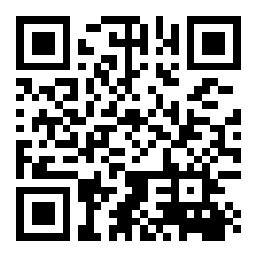