Live Session
Session 10: Graph Learning
Main Track
MMGCL: Meta Knowledge-Enhanced Multi-view Graph Contrastive Learning for Recommendations
Yuezihan Jiang (Kuaishou Technology), Changyu Li (Kuaishou Technology), Gaode Chen (Institute of Information Engineering, Chinese Academy of Sciences, Beijing, China), Peiyi Li (Kuaishou Technology), Qi Zhang (Kuaishou Technology), Jingjian Lin (Kuaishou Technology), Peng Jiang (Kuaishou Inc.), Fei Sun (Chinese Academy of Sciences, Beijing, China) and Wentao Zhang (Peking University)
Abstract
Multi-view Graph Learning is popular in recommendations due to its ability to capture relationships and connections across multiple views. Existing multi-view graph learning methods generally involve constructing graphs of views and performing information aggregation on view representations. Despite their effectiveness, they face two data limitations: Multi-focal Multi-source data noise and multi-source Data Sparsity. The former arises from the combination of noise from individual views and conflicting edges between views when information from all views is combined. The latter occurs because multi-view learning exacerbate the negative influence of data sparsity because these methods require more model parameters to learn more view information. Motivated by these issues, we propose MMGCL, a meta knowledge-enhanced multi-view graph contrastive learning framework for recommendations. To tackle the data noise issue, MMGCL extract meta knowledge to preserve important information from all views to form a meta view representation. It then rectifies every view in multi-learning frameworks, thus simultaneously removing the view-private noisy edges and conflicting edges across different views. To address the data sparsity issue, MMGCL performs meta knowledge transfer contrastive learning optimization on all views to reduce the searching space for model parameters and add more supervised signal. Besides, we have deployed MMGCL in a real industrial recommender system in China, and we further evaluate it on four benchmark datasets and a practical industry online application. Extensive experiments on these datasets demonstrate the state-of-the-art recommendation performance of MMGCL.
Join the Conversation
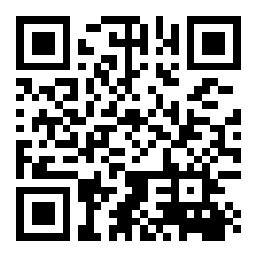