Live Session
Tuesday Posters
Research
MODEM: Decoupling User Behavior for Shared-Account Video Recommendations on Large Screen Devices
Jiang Li (University of Science and Technology of China), Zhen Zhang (Kuaishou Technology Co., Ltd.), Xiang Feng (Kuaishou Technology Co., Ltd.), Muyang Li (Kuaishou Technology Co., Ltd.), Yongqi Liu (Kuaishou Technology Co., Ltd.) and Lantao Hu (Kuaishou Technology Co., Ltd.)
Abstract
In scenarios involving sequence recommendations on large screen devices, such as tablets or TVs, the equipment is often shared among multiple users. This sharing leads to a mixture of behaviors from different users, posing significant challenges to recommendation systems, especially when clear supervisory signals for distinguishing among users are absent. Current solutions tend to either operate in an unsupervised manner or rely on constructed supervisory signals that are not entirely reliable. Moreover, the peculiarities of short video recommendations in this context have not been thoroughly explored in existing research. In response to these challenges, this paper introduces Multi-User Contrastive Decoupling Model (MODEM), a novel short video recommendation model specifically designed for large screen devices. MODEM leverages an attention mechanism, grounded in session segmentation, to disentangle the intertwined user behavior histories. It also discriminates between the impacts of long and short viewing behaviors on short video recommendations by cross-analyzing sequences of both. Furthermore, we have developed a contrastive learning method to oversee the decoupling of user behaviors effectively. Our evaluations demonstrate noticeable improvements through both offline assessments within public datasets and online A/B testing within Kuaishou’s short video recommendation environment on large screen devices. Specifically, our online A/B tests resulted in a 0.55% increase in watch time. These results underscore MODEM’s efficacy in enhancing recommendation quality in shared account contexts.
Join the Conversation
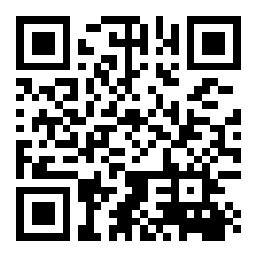