Live Session
Tuesday Posters
Demo
Multi-Preview Recommendation via Reinforcement Learning
Yang Xu (North Carolina State University), Kuan-Ting Lai (Microsoft), Peter Xiong (Microsoft) and Zhong Wu (Microsoft)
Abstract
Preview recommendations serve as a crucial shortcut for attracting users' attention on various systems, platforms, and webpages, significantly boosting user engagement. However, the variability of preview types and the flexibility of preview duration make it challenging to use an integrated framework for multi-preview recommendations under resource constraints. In this paper, we present an approach that incorporates constrained Q-learning into a notification recommendation system, effectively handling both multi-preview ranking and duration orchestration by targeting long-term user retention. Our method bridges the gap between combinatorial reinforcement learning, which often remains too theoretical for practical use, and segmented modules in production, where model performance is typically compromised due to over-simplification. We demonstrate the superiority of our approach through off-policy evaluation and online A/B testing using Microsoft data.
Join the Conversation
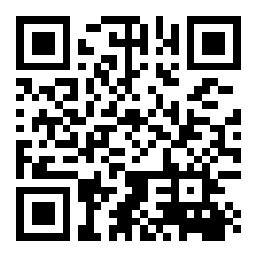