Live Session
Thursday Posters
Industry Poster
Privacy Preserving Conversion Modeling in Data Clean Room
Kungang Li (Pinterest), Xiangyi Chen (Pinterest), Ling Leng (Pinterest), Jiajing Xu (Pinterest), Jiankai Sun (Pinterest) and Behnam Rezaei (Pinterest)
Abstract
In the realm of online advertising, accurately predicting the conversion rates (CVR) is crucial for enhancing advertising efficiency and user satisfaction. However, it faces the challenge that due to user privacy choices and advertiser requirements, the advertising platform cannot get the conversion data from some advertisers, making accurate CVR predictions difficult. Although current methods like split learning or vertical federated learning do not share label information directly, they still exchange sample-level gradients, which introduces a privacy risk as adversaries might infer label information through the shared gradients. To address these challenges, our proposed model training framework incorporates several innovative techniques. Firstly, we employ batch-level aggregated gradients instead of sample-level gradients to enhance privacy. Secondly, to minimize communication costs, we utilize adapter-based parameter-efficient fine-tuning methods such as Low-Rank Adaptation (LoRA) and gradient compression in conversion models. These methods allow for efficient collaboration between different parties while reducing the amount of data transferred. Lastly, we incorporate label differential privacy to protect model privacy. Given that this privacy protection alters the data distribution and can result in significant calibration error, we propose a de-biasing technique to ensure accurate model predictions even with perturbed label information. Our experimental results on industrial datasets demonstrate that our method achieves competitive performance while significantly reducing the communication overhead and complying with advertisers' privacy requirements and user privacy choice. This framework establishes a new benchmark for privacy-preserving and high-performance CVR prediction in the digital advertising industry.
Join the Conversation
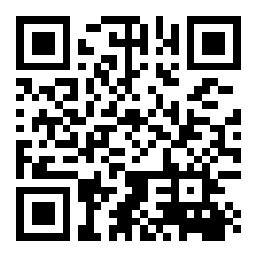