Live Session
Session 6: Multi-task Learning
Main Track
Ranking-Aware Unbiased Post-Click Conversion Rate Estimation via AUC Optimization on Entire Exposure Space
Yu Liu (School of Artificial Intelligence, Nanjing University), Qinglin Jia (Huawei Noah’s Ark Lab), Shuting Shi (Huawei TECHNOLOGIES Co., Ltd), Chuhan Wu (Huawei Noah’s Ark Lab), Zhaocheng Du (Huawei Noah’s Ark Lab), Zheng Xie (Nanjing University), Ruiming Tang (Huawei Noah’s Ark Lab), Muyu Zhang (Huawei TECHNOLOGIES Co., Ltd) and Ming Li (School of Artificial Intelligence, Nanjing University)
Abstract
Estimating the post-click conversion rate(CVR) accurately in ranking systems is crucial in industrial applications. However, this task is often challenged by data sparsity and selection bias, which hinder accurate ranking. Previous approaches to address these challenges have typically focused on either modeling CVR across the entire exposure space or providing unbiased CVR estimation separately. However, the lack of integration between these objectives has limited the overall performance of CVR estimation. Therefore, there is a pressing need for a method that can simultaneously provide unbiased CVR estimates across the entire exposure space. To achieve it, we formulate the CVR estimation task as an Area Under the Curve~(AUC) optimization problem and propose the Entire-space Weighted AUC(EWAUC) framework. EWAUC utilizes sample reweighting techniques to handle selection bias and employs pairwise AUC risk, which incorporates more information from limited clicked data, to handle data sparsity. In order to model CVR across the entire exposure space unbiasedly, EWAUC treats the exposure data as both conversion data and non-conversion data to calculate the loss. The properties of AUC risk guarantee the unbiased nature of the entire space modeling. We provide comprehensive theoretical analysis to validate the unbiased nature of our approach. Additionally, extensive experiments conducted on real-world datasets demonstrate that our approach outperforms state-of-the-art methods in terms of ranking performance for the CVR estimation task. Our source code is available at https://anonymous.4open.science/r/EWAUC-C40D.
Join the Conversation
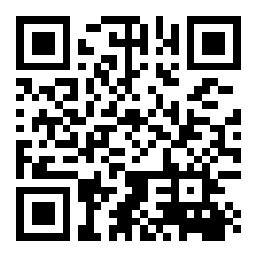