Live Session
Session 12: Optimisation and Evaluation 2
Main Track
Right Tool, Right Job: Recommendation for Repeat and Exploration Consumption in Food Delivery
Jiayu Li (Tsinghua University), Aixin Sun (Nanyang Technological University), Weizhi Ma (Tsinghua University), Peijie Sun (Hefei University of Technology, School of Computer and Information) and Min Zhang (Tsinghua University)
Abstract
From e-commerce to music and news, recommender systems are tailored to specific scenarios. While researching generic models applicable to various scenarios is crucial, studying recommendations based on the unique characteristics of a specific and vital scenario holds both research and, more importantly, practical value.In this paper, we focus on store recommendations in the food delivery scenario, which is an intriguing and significant domain with unique behavior patterns and influential factors. First, we offer an in-depth analysis of real-world food delivery data across platforms and countries, revealing that (i) repeat and exploration orders are both noticeable behaviors and (ii) the influences of historical and collaborative situations on repeat and exploration consumption are distinct.Second, based on the observations, we separately design two simple yet effective recommendation models: \textit{RepRec} for repeat orders and \textit{ExpRec} for exploration ones. An ensemble module is further proposed to combine recommendations from two models for a unified recommendation list. Finally, experiments are conducted on three datasets spanning three countries across two food delivery platforms. Results demonstrate the superiority of our proposed recommenders on repeat, exploration, and combined recommendation tasks over various baselines. Such simple yet effective approaches will be beneficial for real applications. This work shows that dedicated analyses and methods for domain-specific characteristics are essential for the recommender system studies.
Join the Conversation
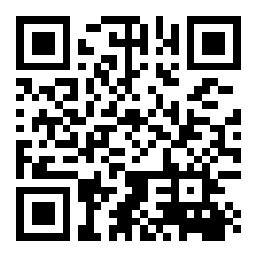