Live Session
Wednesday Posters
Research
Scene-wise Adaptive Network for Dynamic Cold-start Scenes Optimization in CTR Prediction
Wenhao Li (Huazhong University of Science and Technology), Jie Zhou (Beihang University), Chuan Luo (Beihang University), Chao Tang (Meituan), Kun Zhang (Meituan) and Shixiong Zhao (The University of Hong Kong)
Abstract
In the realm of modern mobile E-commerce, providing users with nearby commercial service recommendations through location-based online services has become increasingly vital. While machine learning approaches have shown promise in multi-scene recommendation, existing methodologies often struggle to address cold-start problems in unprecedented scenes: the increasing diversity of commercial choices, along with the short online lifespan of scenes, give rise to the complexity of effective recommendations in online and dynamic scenes. In this work, we propose Scene-wise Adaptive Network (SwAN), a novel approach that emphasizes high-performance cold-start online recommendations for new scenes. Our approach introduces several crucial capabilities, including scene similarity learning, user-specific scene transition cognition, scene-specific information construction for the new scene, and enhancing the diverged logical information between scenes. We demonstrate SwAN's potential to optimize dynamic multi-scene recommendation problems by effectively online handling cold-start recommendations for any newly arrived scenes. More encouragingly, SwAN has been successfully deployed in Company M's online catering recommendation service, which serves millions of customers per day, and SwAN has achieved a 5.64% CTR index improvement relative to the baselines and a 5.19% increase in daily order volume proportion.
Join the Conversation
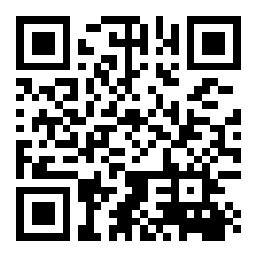