Live Session
Thursday Posters
Industry Poster
Sliding Window Training - Utilizing Historical Recommender Systems Data for Foundation Models
Swanand Joshi (Netflix), Yesu Feng (Netflix), Ko-Jen Hsiao (Netflix), Zhe Zhang (Netflix) and Sudarshan Lamkhede (Netflix)
Abstract
Long-lived recommender systems (RecSys) often encounter lengthy user-item interaction histories that span many years. To effectively learn long term user preferences, Large RecSys foundation models (FM) need to encode this information in pretraining. Usually, this is done by either generating a long enough sequence length to take all history sequences as input at the cost of large model input dimension or by dropping some parts of the user history to accommodate model size and latency requirements on the production serving side. In this paper, we introduce a sliding window training technique to incorporate long user history sequences during training time without increasing the model input dimension. We show the quantitative \& qualitative improvements this technique brings to the RecSys FM in learning user long term preferences. We additionally show that the average quality of items in the catalog learnt in pretraining also improves.
Join the Conversation
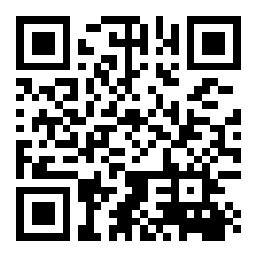