Live Session
Session 3: Bias and Fairness 2
Main Track
The Fault in Our Recommendations: On the Perils of Optimizing the Measurable
Omar Besbes (Columbia University), Yash Kanoria (Columbia University) and Akshit Kumar (Columbia University)
Abstract
Recommendation systems play a pivotal role on digital platforms by curating content, products and services for users. These systems are widespread, and through customized recommendations, promise to match users with options they will like. To that end, data on engagement is collected and used, with, e.g., measurements of clicks, but also purchases or consumption times. Most recommendation systems are ranking-based, where they rank and recommend items based on their predicted engagement. However, the engagement signals are often only a crude proxy for user utility, as data on the latter is rarely collected or available. This paper explores the following critical research question: By optimizing for measurable proxies, are recommendation systems at risk of significantly under-delivering on user utility? If that is indeed the case, how can one improve utility which is seldom measured? To study these questions, we introduce a model of repeated user consumption in which, at each interaction, users select between an outside option and the best option from a recommendation set. Our model accounts for user heterogeneity, with the majority preferring “popular” content, and a minority favoring “niche” content. The system initially lacks knowledge of individual user preferences but can learn these preferences through observations of users’ choices over time. Our theoretical and numerical analysis demonstrate that optimizing for engagement signals can lead to significant utility losses. Instead, we propose a utility-aware policy that initially recommends a mix of popular and niche content. We show that such a policy substantially improves utility despite not measuring it. In fact, in the limit of a forward-looking platform with discount factor $\delta \to 1$, our utility-aware policy achieves the best of both worlds: near-optimal user utility and near-optimal engagement simultaneously. Our study elucidates an important feature of recommendation systems; given the ability to suggest multiple items, one can perform significant exploration without incurring significant reductions in short term engagement. By recommending high-risk, high-reward items alongside popular items, systems can enhance discovery of high utility items without significantly affecting engagement.
Join the Conversation
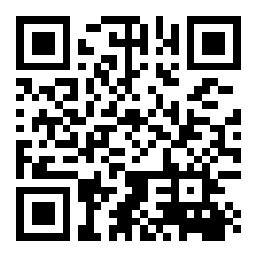