Live Session
Tuesday Posters
Research
Towards Green Recommender Systems: Investigating the Impact of Data Reduction on Carbon Footprint and Algorithm Performances
Giuseppe Spillo (University of Bari), Allegra De Filippo (DISI Università di Bologna), Cataldo Musto (Dipartimento di Informatica - University of Bari), Michela Milano (DISI Universita' di Bologna) and Giovanni Semeraro (University of Bari)
Abstract
This work investigates the path toward green recommender systems by examining the impact of data reduction on both model performance and carbon footprint. In the pursuit of developing energy-efficient recommender systems, we investigated whether and how reducting training data impacts the performances of several representative recommendation models. In order to obtain a fair comparison, all the models were run based on the implementations available in a popular recommendation library, i.e., RecBole, and used the same experimental settings. Results indicate that: (a) data reduction can be a promising strategy to make recommender systems more sustainable at the cost of a lower accuracy; (b) training recommender systems with less data makes the suggestions more diverse and less biased. Overall, this study contributes to the ongoing discourse on the development of recommendation models that meet the principles of SDGs, laying the groundwork for the adoption of more sustainable practices in the field.
Join the Conversation
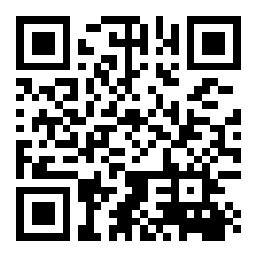