Live Session
Session 16: Large Language Models 2
Main Track
Unleashing the Retrieval Potential of Large Language Models in Conversational Recommender Systems
Ting Yang (Hong Kong Baptist University) and Li Chen (Hong Kong Baptist University)
Abstract
Conversational recommender systems (CRSs) aim to capture user preferences and provide personalized recommendations through interactive natural language interactions. The recent advent of large language models (LLMs) has revolutionized human engagement in natural conversations, driven by their extensive world knowledge and remarkable natural language understanding and generation capabilities. However, introducing LLMs into CRSs presents new technical challenges. Specifically, directly prompting LLMs for recommendation generation requires understanding a large and evolving item corpus, as well as grounding the generated recommendations in the real item space. Moreover, generating recommendations based on external recommendation engines or directly integrating their suggestions into responses may constrain the overall performance of LLMs, since these engines generally have inferior representation abilities compared to LLMs. To address these challenges, we propose an end-to-end large-scale CRS model, ReFICR, a novel LLM-enhanced conversational recommender that empowers a retrievable large language model to perform CRS subtasks by following retrieval and generation instructions through lightweight tuning. By decomposing the complex CRS task into multiple subtasks, we formulate these subtasks into two types of instruction formats: retrieval and generation. The hidden states of ReFICR are utilized as text embeddings to represent user preferences and items for retrieval. Simultaneously, ReFICR is trained to handle generative tasks. We optimize the contrastive objective to enhance text embeddings for retrieval and jointly fine-tune the large language model objective for generation. Our experimental results on public datasets demonstrate that ReFICR significantly outperforms baselines in terms of recommendation accuracy. Our code is publicly available at the link: https://anonymous.4open.science/r/ReFICR-0C3A.
Join the Conversation
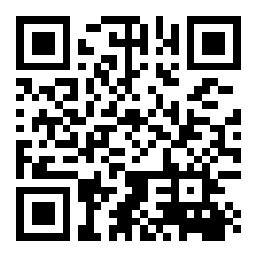